In the landscape of technology platforms of the future, the role of artificial intelligence (AI) has become increasingly prominent. It continues to revolutionize various industries and transform the way we live and work.
I witness this remarkable shift from the first-row seats of transformative technology delivery, and I find myself continuously surprised by the immense potential AI holds in technology delivery management.
With each passing day, AI continues to redefine the boundaries of what is possible, empowering businesses to streamline their operations and deliver interesting results.
The benefits go from optimizing wide areas of company processes and resource allocation to automating repetitive tasks. It goes as far as even predicting potential bottlenecks. AI has already proven to be an important asset in driving the success of companies.
Unleashing AI’s Potential in Technology Delivery Management
One of AI’s biggest advantages is its ability to analyze large amounts of data very quickly. AI also uses machine learning algorithms to extract insights from complex datasets.
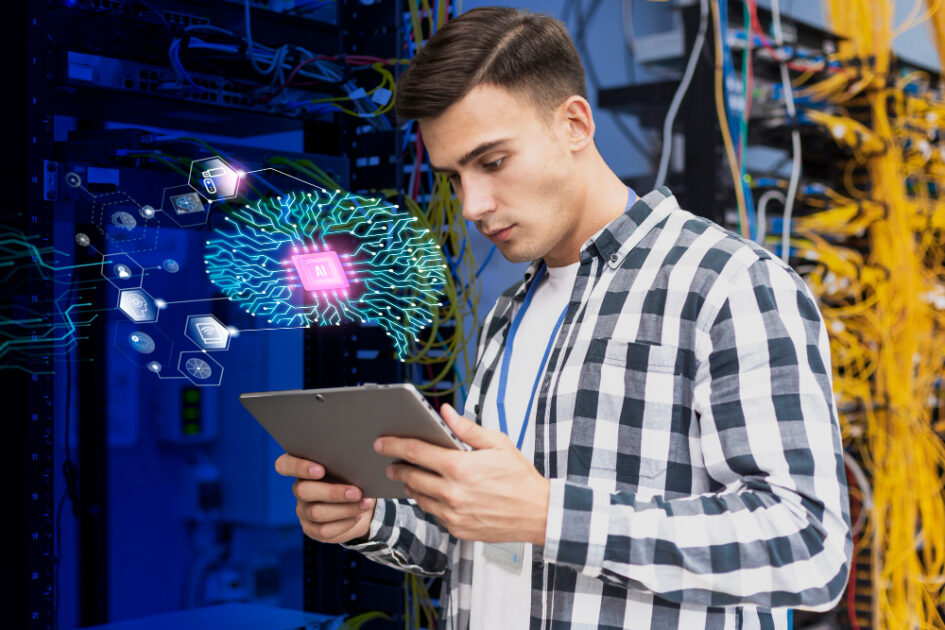
Companies can make significant decisions based on that data. With AI implemented into the system platform, they have a powerful tool to proactively identify and mitigate risks during the project timeline.
Predictive Insights Powered by AI and Data Lakes
AI’s predictive capabilities have redefined the way you might approach (any kind of) resource allocation.
For example, let’s imagine you have only a limited amount of people capable of servicing your device infrastructure. However, the infrastructure itself is exceptionally complex (it can cover multiple countries).
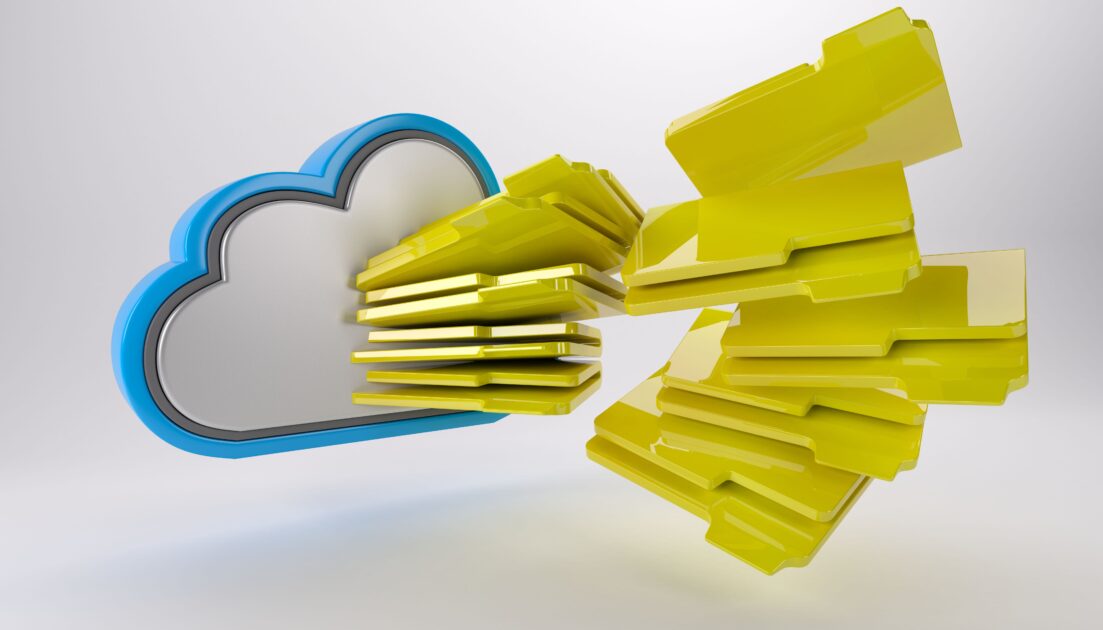
Then you need to decide well where the people will be sent for maintenance if you want to ensure reliable infrastructure operation. In some cases, you need to fulfill government regulations regarding the reliability of your assets, which restricts you even further.
This is where data lake with AI comes into play. AI generates large amounts of data in real-time and creates organized structures called data lakes. Data lake is the ultimate source of truth for any predictions or conclusions. You can let AI analyze this data lake and historical data and patterns inside.
The result of that is the exact forecast of where to send service resources at which time. Such usage of data lake, of course, reduces the costs of people’s work but also ensures that the right resources are available at the right time.
Collaborative Synergy: Humans and AI in Harmony
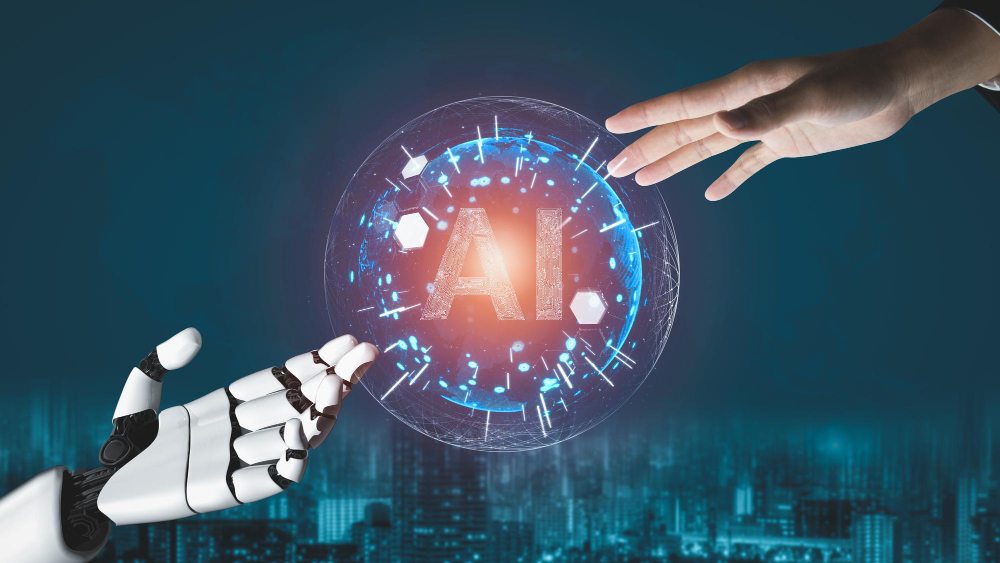
It is important to note that AI is not meant to replace human expertise. You still need to implement the back door into your system so that you can easily verify and correct the results of such AI processing. The cooperation between humans and AI is still needed. AI might excel at processing large amounts of data, but it completely lacks human intuition and creativity
The collaboration between humans and AI creates a balance where each complements the strengths of the other.
Revolutionizing Utility Infrastructure Maintenance with AI
Let’s stay with our electricity utility infrastructure case. Maintaining such infrastructure is a critical task that covers the smooth functioning of several devices on each electricity pole, and you can have potentially thousands of such poles.
Fortunately, the convergence of drone photography and AI machine learning models that are now possible to implement inside software solutions and processes has opened up new possibilities, changing forever the way we approach utility infrastructure maintenance.
Drones equipped with high-resolution cameras can capture detailed images of utility infrastructure at any time during the day, including power lines, pipelines, and communication towers.
These photographs provide a comprehensive view of the infrastructure, allowing for a more accurate assessment of its condition. You can leverage AI machine learning models to analyze and process the pictures to extract valuable insights, leading to improved maintenance practices.
Detecting Anomalies and Predicting Problems with AI
A key advantage of using machine learning models with drone photography is they can reliably detect and predict potential issues coming from the devices in the pictures. Sure, a dedicated, trained person would do just that as well, but not in such a short time. The only thing you need to do is to make sure you have well-trained machine-learning models.
For example, the models can detect signs of corrosion or structural damage. If your software platform can identify these issues early on, maintenance teams can take proactive measures to address them soon.
Creating Digital Twins for Real-time Infrastructure Monitoring
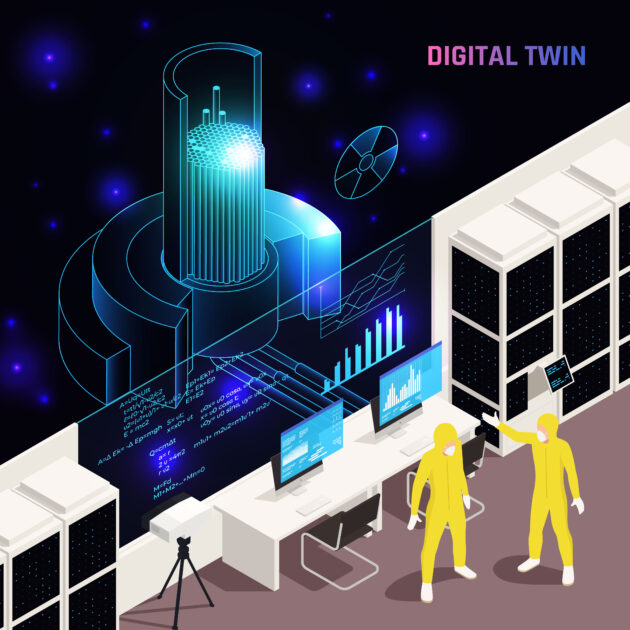
The benefit of combining drone photography with AI is the ability to create a digital twin of the utility infrastructure. You can generate a digital replica of the infrastructure by continuously capturing images and feeding them into the AI models.
This virtual representation provides a real-time view of the infrastructure’s condition. It allows the maintenance teams to monitor changes and plan maintenance activities more effectively. The digital twin also serves as a valuable resource for training AI models further, enhancing their accuracy and predictive capabilities over time.
AI’s Reshaping of Delivery Processes: Key Impacts
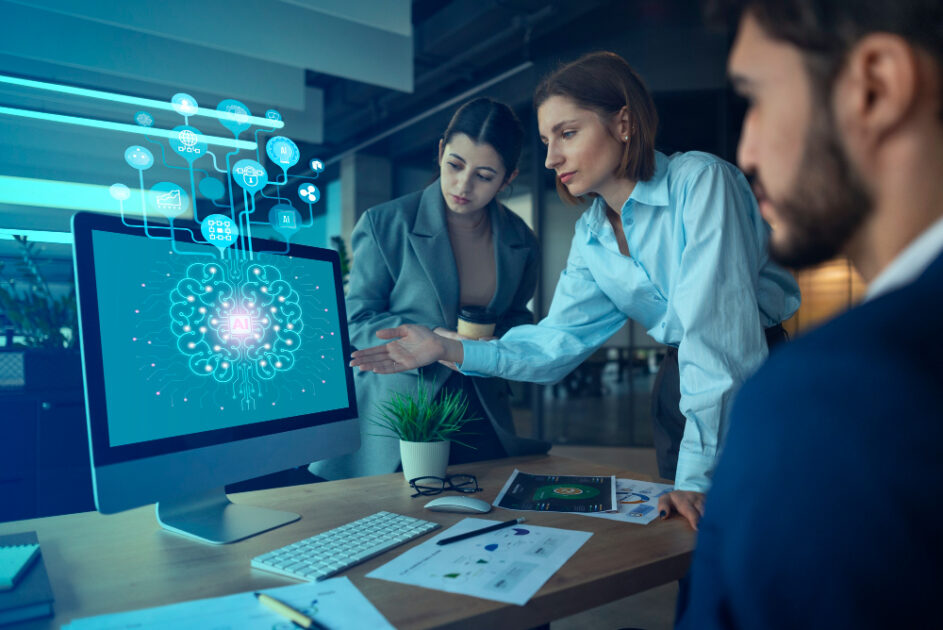
The implementation of AI in technology delivery processes significantly changes the way projects are planned, executed, and managed. Here are some key impacts of such AI implementation that you can notice in your case as well.
Enhanced Efficiency
If you happen to have many repetitive and time-consuming tasks in your software processes, AI automatizes that and allows teams to focus on more complex and strategic activities instead. This alone leads to increased efficiency and productivity in your processes.
Well-trained AI algorithms can analyze your data on the backside and identify repeating patterns. Then, AI uses them to make predictions going forward.
Improved Accuracy
AI algorithms can process and analyze data with a high level of accuracy. In the case of machine learning model processing, we speak here about the probability of 99% and higher that the conclusions AI makes are correct. This level of accuracy is already possible today. This reduces the chances of human error and improves the overall quality of technology delivery.
Streamlining Project Management with AI
The implementation of AI also streamlines project management by automating various processes. Today, you will find many project management software tools with native support of AI in different forms. We don’t need to go too far away and just take Microsoft Outlook or Edge as an example. ChatGPT input is already there and ready to use. AI really can today assist in project planning and scheduling.
Proactively Identifying Issues in Tech Delivery with AI
What about proactive detecting and predicting potential issues or bottlenecks in technology delivery processes? Yes, AI can do that as well. By analyzing data, AI can alert teams to potential risks. This helps prevent delays and ensures smooth project execution.
Data-Driven Decision-Making in Technology Delivery
You can make much easier data-driven decisions by involving AI implementation in your technology platform. First, AI quickly generates a large amount of data, which would be quite useless if there were no effective way how to analyze it quickly. But AI algorithms can extract valuable insights fast and provide actionable recommendations.
Achieving Continuous Improvement with AI
AI implementation gives you the possibility of continuous learning and improvement. AI algorithms can analyze historical data and adapt to changing circumstances. This can help you to refine processes and enhance overall performance in technology delivery.
Implementing AI: Step-by-Step
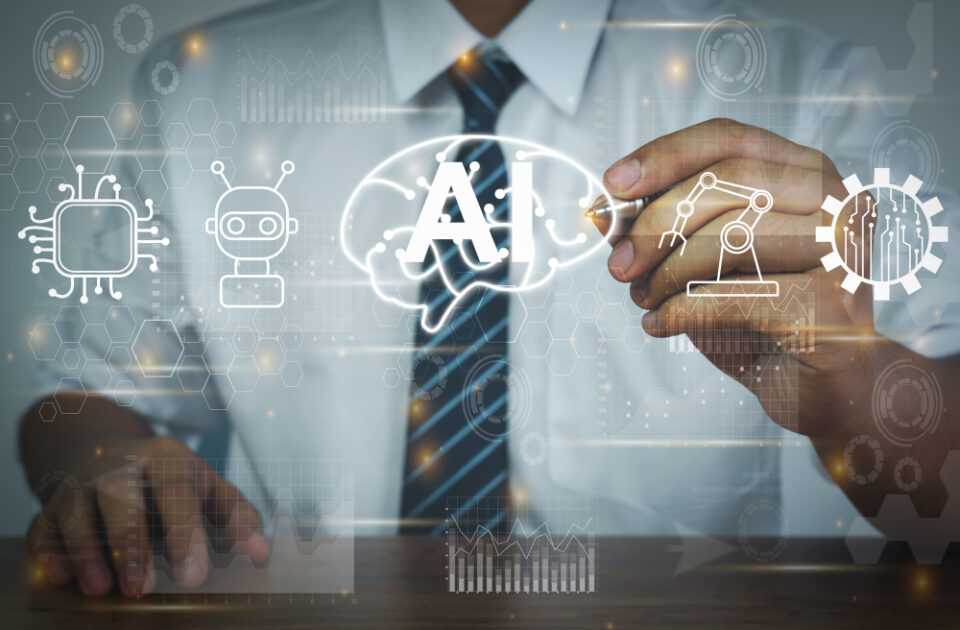
Let’s try to answer now the question of what’s the best way to approach such implementation. Say, if you are just about to start with all that. It can be overwhelming and confusing. Here is a simple guidance for you to narrow the chaos into some clear concentration points.
#1. Identify the Use Case
Start with identifying specific use cases within the project where AI models can add value. Work with the project team to understand their pain points and areas where AI can provide insights or automate tasks.
Only by focusing on specific use cases can you come up with the implementation of AI models that really address the team’s needs.
#2. Data Preparation and Feature Engineering
Data preparation is the most important step in implementing AI models. You will need to make sure you have enough relevant data to start training the machine learning models.
Work closely with your team’s data scientists to gather and preprocess relevant data. This may involve cleaning, transforming, and aggregating data to make it suitable for AI model training.
#3. Model Selection and Training
Select the appropriate AI models based on the requirements and available data. Pick the most suitable out of the offering of cloud vendor of your choice, as the easiest route.
Collaborate with data scientists to train the chosen models using the already prepared data. This involves feeding the models with labeled or historical data to learn patterns. Regularly evaluate and fine-tune the models to improve their performance over time, as the data might change.
#4. Integration with Project Workflows
Integrate the AI models (natively, if possible) into the project team’s workflows. This may involve developing APIs or interfaces to interact with the AI models. If you are already on a cloud platform (like Amazon Web Services, for example), such integration comes quite naturally.
Ensure that the integration is user-friendly. Align it with the existing project management tools and processes. This will speed up the adoption and utilization of AI models by the project team.
#5. Continuous Monitoring and Feedback
Continuously monitor the performance of the AI models and gather feedback from the project team. Encourage the team to provide feedback on the usefulness and usability of the AI models in their day-to-day work.
This feedback loop will help identify areas for improvement and ensure that the AI models remain aligned with the project team’s needs.
Success Stories: AI in Real-World Technology Delivery
Here are some real-world technology delivery processes (additionally to the electricity infrastructure example above) where AI was successfully implemented in technology delivery projects. We’ll also highlight what was the actual benefit of such AI implementation.
AI-Powered Chatbots for Customer Support
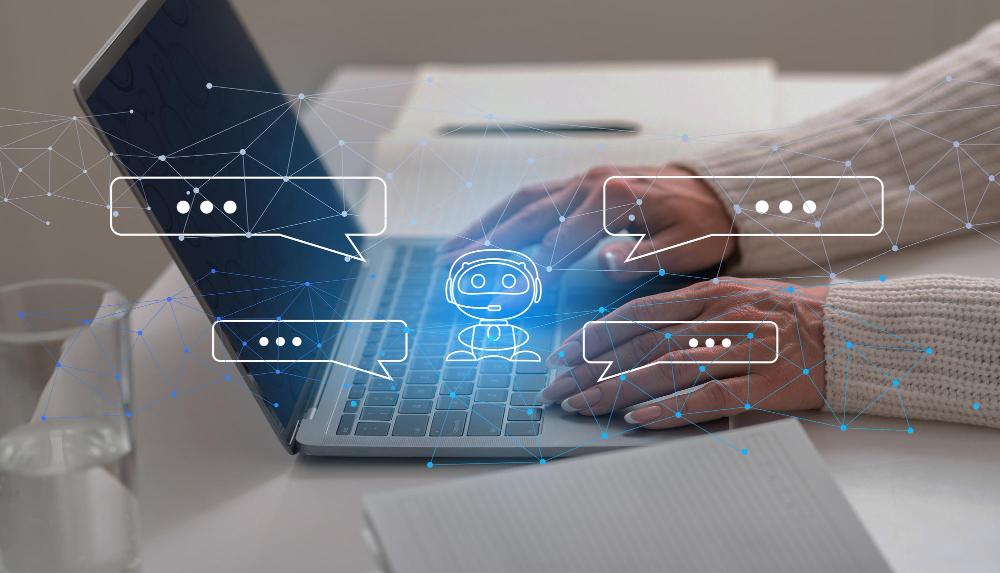
AI-powered chatbots have been deployed in various industries to handle customer inquiries and support. These chatbots use natural language processing and machine learning to understand and respond to customer queries. The benefit is improved customer service with 24/7 availability, faster response times, and reduced workload for human support agents.
Fraud Detection in Financial Services
AI algorithms can analyze financial data and identify patterns of suspicious activities. Automation of such detection is possible, and AI can flag such transactions in real-time. As a result, it minimizes financial losses and protects customers from such financially dangerous activities.
Autonomous Vehicles and Road Safety
AI plays is already part of the development of autonomous vehicles. Machine learning algorithms enable vehicles to understand their surroundings and use them for safe navigation.
The benefit is the potential of safer roads (eliminating skills unreliability of some parts of humanity, as the ever-growing road accident statistics prove to us every day).
Personalized Recommendations in E-commerce
E-commerce solutions have a platform where AI algorithms can analyze user behavior or purchase history to provide personalized product recommendations. This enhances the customer experience and increases sales as a side effect. It also offers relevant and tailored suggestions for users.
Real-World Use Cases: AI’s Tangible Impact on Delivery Management
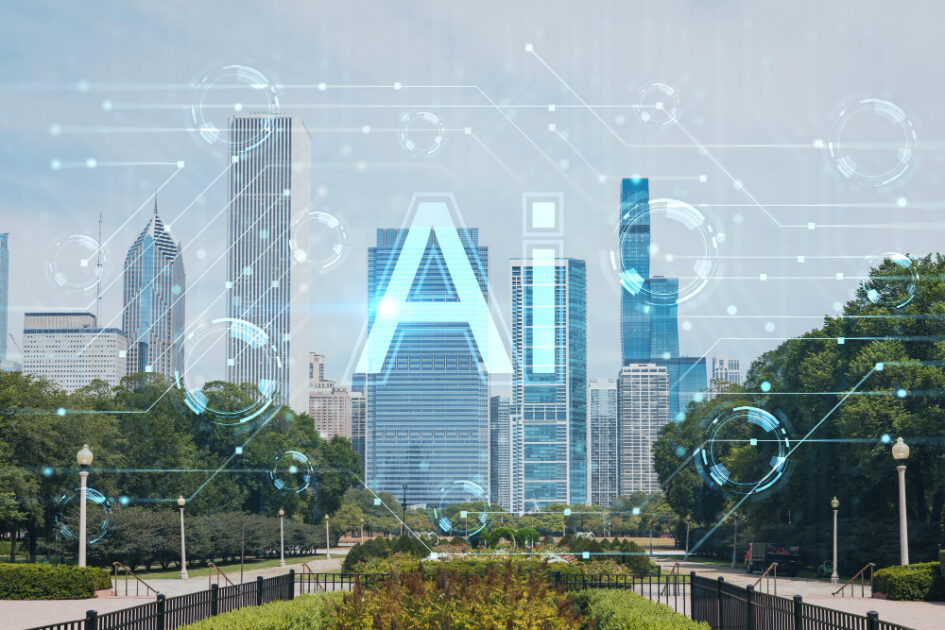
We can now jump to some of the practical use cases and companies that have already started with AI, and they have tangible success stories to share.
#1. Netflix: Personalizing Content Recommendations
Netflix leverages AI models in its recommendation system to personalize content suggestions for its users. It analyzes user viewing habits, preferences, and ratings and then uses AI algorithms to generate personalized recommendations to improve user engagement and retention.
The main benefit for Netflix is increased user satisfaction and longer viewing sessions, leading to higher subscription rates.
#2. Salesforce: Empowering Sales and Marketing with AI
The main benefit in this case was the improvement of customer engagement and the drive of revenue growth.
#3. IBM Watson: Transforming Healthcare Decision-Making
IBM Watson is an AI-powered platform that has been successfully implemented across various industries. One notable use case is healthcare, where Watson analyzes medical data, assists in diagnosis, and provides treatment recommendations.
The main benefit is improved accuracy and efficiency in healthcare decision-making, leading to better patient outcomes and reduced healthcare costs.
#4. Bringg: Optimizing Delivery Routes and Schedules
Bringg is a delivery and fulfillment orchestration platform that uses AI to optimize delivery routes and schedules. Its AI models analyze various factors, such as traffic patterns, weather conditions, and order volumes, to dynamically optimize delivery operations.
The main benefit for Bringg’s clients is increased operational efficiency, reduced delivery costs, and improved customer satisfaction.
#5. DispatchTrack: Automating Last-Mile Delivery
DispatchTrack is a delivery management software company that uses AI to automate and optimize last-mile delivery operations. Their AI models analyze real-time data, including driver availability, traffic conditions, and delivery preferences. They can dynamically assign and optimize delivery routes.
The main benefit for DispatchTrack’s clients is improved delivery efficiency, reduced fuel costs, and enhanced customer experience.
#6. Onfleet: Streamlining Delivery Operations
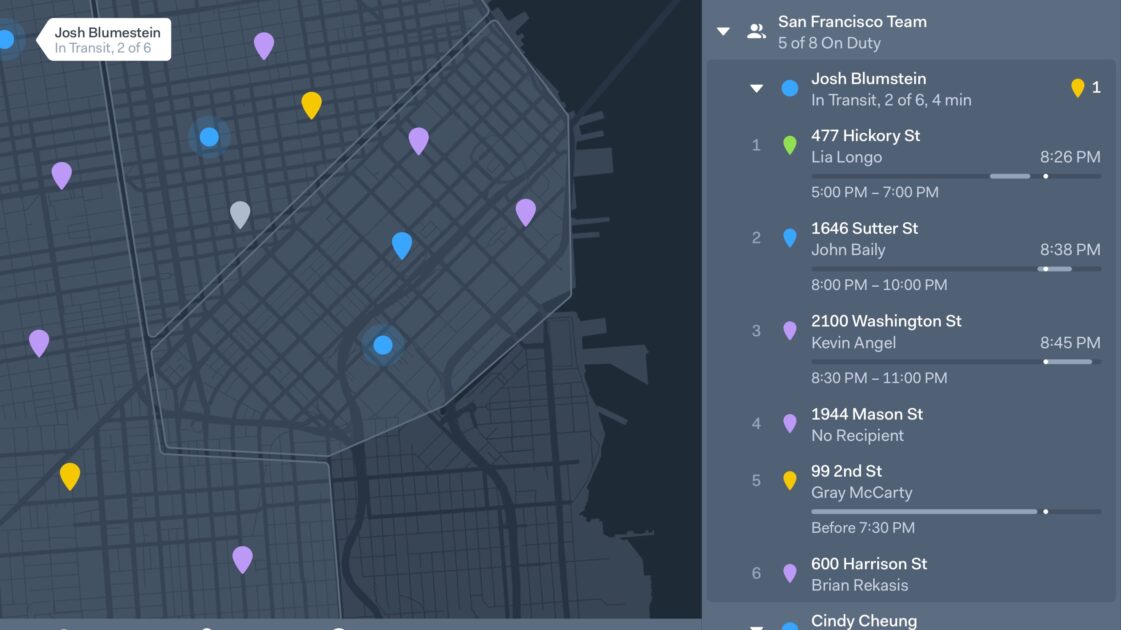
Onfleet is a delivery management platform that uses AI to streamline and optimize delivery operations. Their AI models analyze historical data and real-time information to predict delivery times and optimize routes.
The main benefit for Onfleet’s clients is improved delivery efficiency, reduced operational costs, and enhanced visibility and control over the delivery process.
#7. LogiNext: Enhancing Logistics Efficiency
LogiNext is a logistics optimization software company that uses AI to optimize delivery routes and schedules. Their AI models analyze various factors, such as traffic patterns, delivery constraints, and customer preferences, to generate optimal routes and schedules.
The main benefit for LogiNext’s clients is improved delivery efficiency, reduced transportation costs, and enhanced customer satisfaction.
#8. Circuit: Optimizing Multi-Stop Delivery Routes
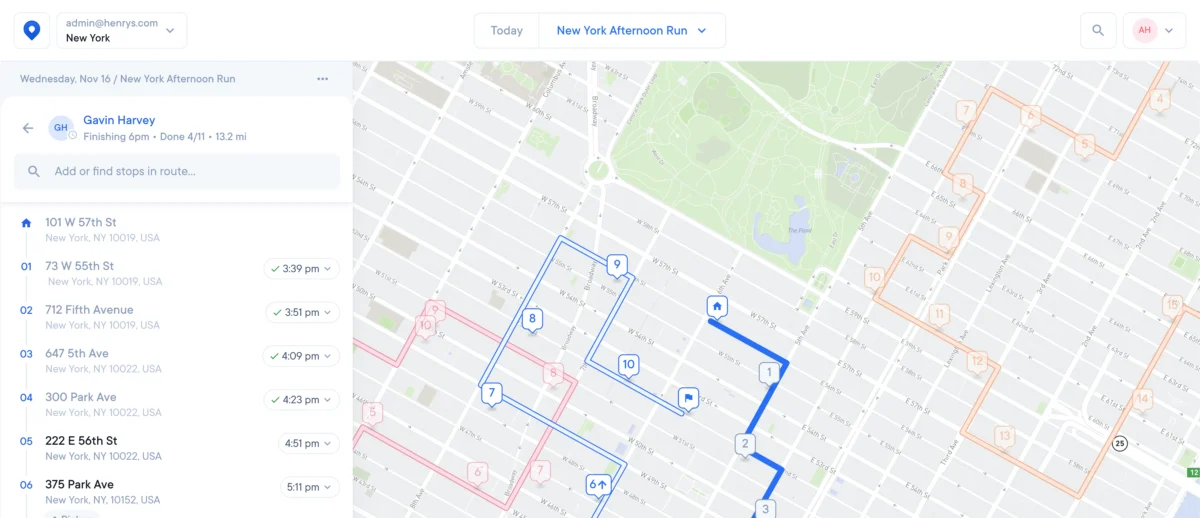
Circuit is a delivery route planning software that uses AI to optimize delivery routes for multiple stops. Their AI models analyze various factors, such as delivery time windows, traffic conditions, and vehicle capacities, to generate efficient routes.
The main benefit for Circuit’s clients is reduced mileage, improved delivery time accuracy, and increased driver productivity.
Conclusion
Technology delivery will be even more dependent on AI implementations of various types going forward. This trend already started, and many big worldwide companies have that now as one of the top three priorities of the upcoming fiscal year. The sooner we start having hands-on with such technologies, the more relevant we will be in the market.