Meta’s Self-Taught Evaluator That Could Redefine AI Development

Meta’s new “Self-Taught Evaluator” can improve AI models without human feedback, making AI training faster and easier. This could accelerate AI development and change how AI is used in businesses. Traditionally, AI training relies on human feedback, which is time-consuming and costly. Meta’s innovation addresses the challenge of constantly updating training data, speeding up the process and reducing costs.
Enter Meta’s Self-Taught Evaluator
Meta’s “Self-Taught Evaluator” solves these issues by removing the need for human feedback entirely. It allows AI to generate its own tasks, evaluate its own performance, and adjust its strategies autonomously, creating a closed-loop system where AI teaches itself to improve without external input.
The self-taught evaluator uses a technique called “Chain of Thought” reasoning, enabling the AI to break down complex tasks into smaller, more manageable steps. This method proves highly effective in areas like mathematics, scientific analysis, and coding. The AI generates possible solutions to a problem, then evaluates them based on accuracy, efficiency, and creativity, adjusting its internal models accordingly.
The Power of AI-Generated Data
A critical aspect of Meta’s self-taught evaluator is its reliance on fully AI-generated data. Rather than using human-labeled data, the AI model creates its own synthetic tasks and responses, which it then judges using a large language model (LLM) as a “judge.” This repetetive process enables the model to improve its accuracy over time.
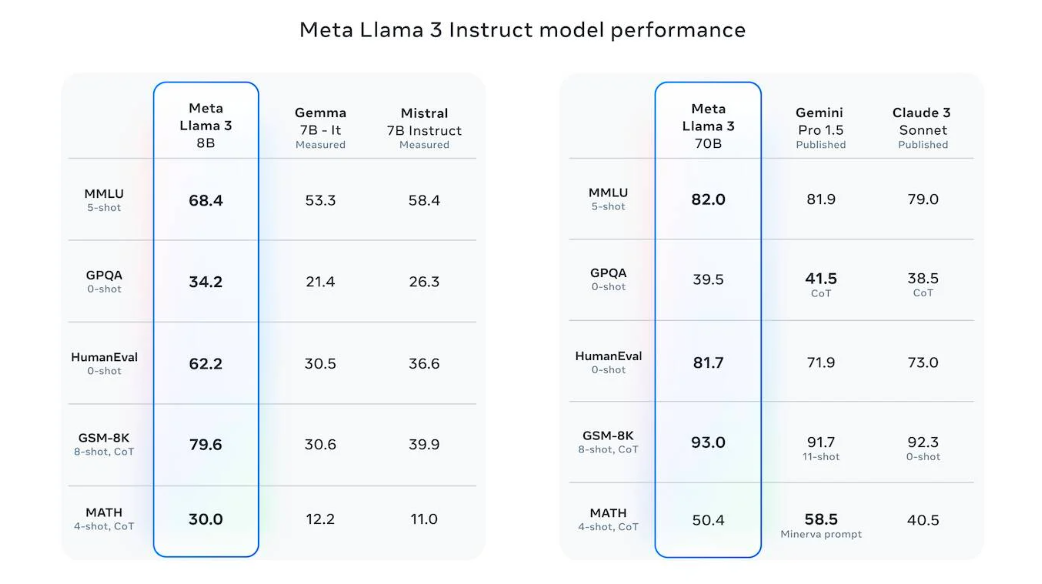
One of the most impressive achievements of the self-taught evaluator is its impact on Meta’s LLaMA 3 70B instruct model. After several iterations, the self-taught evaluator improved the model’s accuracy on the Reward Bench benchmark from 75.4% to 88.3%. In some cases, the model outperforms reward models that rely on human-labeled data. Using a majority vote system, accuracy can reach up to 88.7%.
Real-World Applications
Meta’s self-taught evaluator is already being applied to real-world tasks. For example, it has shown significant advancements in Reward Bench, a benchmark designed to test how well models align with human preferences. Reward models are crucial in areas like safety, ethical decision-making, and multi-step reasoning tasks.
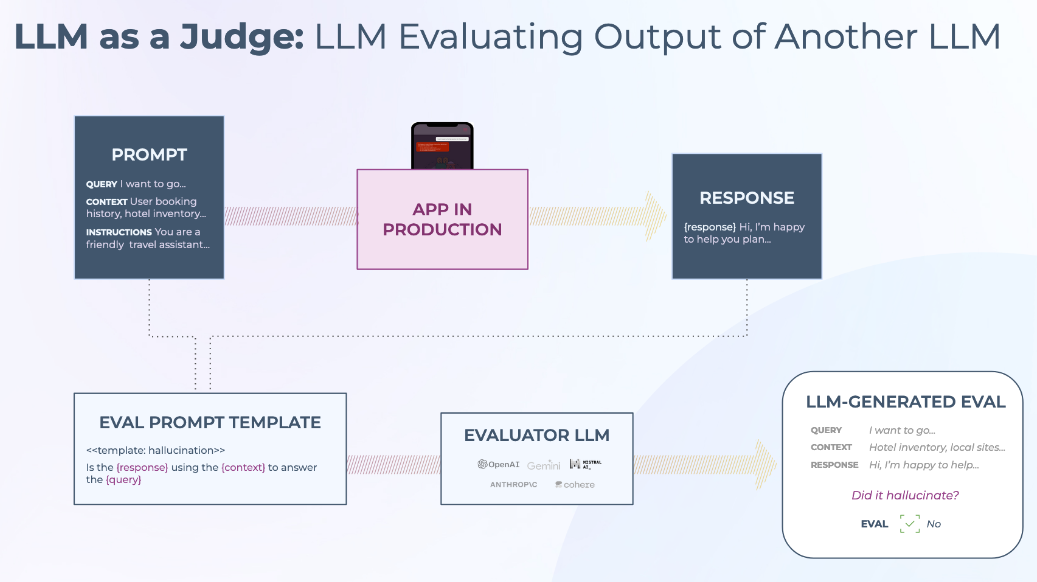
The shift to synthetic data offers several advantages beyond speed and efficiency. Human feedback models often lag behind the generation of new data, slowing the training process. With the self-taught evaluator, this lag is eliminated, allowing the AI to generate, evaluate, and learn in real time.
Automating the evaluation process also helps eliminate human bias. Human evaluators introduce subjectivity in understanding tone, context, or cultural nuance. Meta’s self-taught evaluator ensures consistent standards, making it valuable for global applications that require adaptation to different languages and cultural contexts.
Expanding the Horizon: Meta’s Other AI Innovations
Meta’s advancements don’t stop with the self-taught evaluator. The company has also updated its Segment Anything Model (SAM) to version 2.1. SAM 2.1 improves image and video segmentation, making it easier to isolate objects in complex visual environments. This model has been downloaded over 700,000 times and is used in fields like medical imaging and meteorology.
SAM 2.1 is better at handling small and visually similar objects. This is important for applications like autonomous driving and robotics. New data augmentation techniques help SAM simulate objects in different scenarios. This improves SAM’s ability to deal with occlusions, where objects are partially hidden.
Meta has also launched a developer suite allowing users to fine-tune SAM with their own datasets, facilitating more customization and innovation in visual segmentation.
On the language side, Meta has been advancing MetaLM, an open-source language model designed to generate natural-sounding speech that reflects different emotions. From excitement to surprise, MetaLM allows developers to create more expressive AI-driven conversations. By making MetaLM open-source, Meta has empowered developers to customize and enhance the model, further driving innovation in speech-to-text and text-to-speech technologies.
The Future of AI Training
Meta’s innovations are leading to a new era of autonomous AI systems.These systems can automate complex tasks and reduce human bias. They are faster, smarter, and more scalable. Advancements like LLaMA, SAM, and MetaLM are pushing the boundaries of AI. Meta is setting the stage for a future where AI can improve itself independently. This will transform industries and provide even greater support.