Image annotation is the base of efficient labeling to train machine learning models easily. Check out these best image annotation tools to make your process effective.
What is Image Annotation?
A machine learning model processes tagged images after the manual annotation is finished to reproduce the annotations without human oversight. Any label errors are also duplicated because image annotation establishes the criteria the model strives to follow.
Labeling or categorizing an image with descriptive data that aids in identifying and classifying objects, persons, or scenes within the picture are an image annotation.
Image annotation is crucial in disciplines like computer vision, robotics, and autonomous driving because it enables robots to comprehend and interpret visual data.

Drawing boundary boxes around things in a picture, labeling objects with text, or dividing an image into sections based on their visual features are examples of image annotation.
Benefits of using Image Annotation
Many industries, including e-commerce, healthcare, and autonomous driving, can profit from image annotation. It makes it possible for machines to correctly identify and categorize objects in images. This enhances their capacity to perform activities like object detection, image search, and diagnosis.
Increased productivity, efficiency, and cost savings may result from this. Annotated photos can also train and enhance machine learning models, improving their precision and effectiveness. The ability of machines to comprehend and interpret visual data is made possible by picture annotation, which advances the science of computer vision.
Use cases of Image Annotation

Image Annotation is highly useful with advancements happening in the technological sector. Where everything revolves around data, it’s also essential to label it right for models to understand it better.
Now, let us look through some of the use cases of image annotation.
Object Detection
It is the method of locating and identifying particular items inside a picture. An algorithm can recognize the objects in a photo by using image annotation to label them. This is helpful in applications like self-driving cars, which must realize and steer clear of oncoming traffic.
Image Classification
The process of placing a picture into one of many predetermined categories is known as image categorization. The right category can be assigned to the image via image annotation. Applications like content moderation, where photos must be categorized as appropriate or inappropriate, can benefit from this.
Medical Image Analysis
Labels showing the presence of cancers, fractures, or other anomalies can be added to medical pictures like X-rays or MRIs. This facilitates better picture analysis and enhances the diagnostic precision of medical professionals and researchers.
Although labor-intensive, automating inventory control and surveillance with image processing is worthwhile. The annotation of images is a crucial component of agile security.
It supports operations like pedestrian tracking, facial recognition for theft detection, thermal vision, night vision during the darkest hours, crowd detection, etc. You can solve complex projects without hassle with enhanced access to advanced tech.
Let us explore some of the top tools that can be used for image annotation for efficient labeling.
Markup Hero
You can instantly share your photographs and files with Markup Hero while adding free annotations in any web browser. It’s straightforward to use and simple to learn.
It is an effective tool for picture annotation that enables users to quickly add different shapes, arrows, text, and other components to photographs. Users can work with others in real-time and communicate visually because of the tool’s user-friendly design.
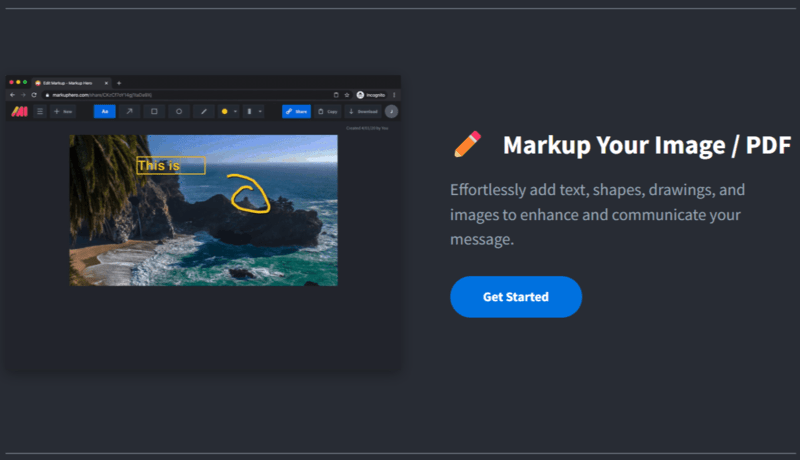
With Markup Hero, you can annotate photographs in various ways, such as emphasizing crucial information, calling attention to specific regions, adding labels and descriptions, and more. Users of the program may also crop, resize, and rotate pictures, making it simple to get the perfect shot.
Users can send and receive comments and feedback on annotated photographs with ease. Altogether, Markup Hero is a simple, adaptable, and robust image annotation tool perfect for anyone wishing to interact with others in real time and communicate graphically.
Keylabs
With the help of Keylabs, users can annotate photos by adding bounding boxes, key points, and semantic segmentation. AI developers can annotate images more efficiently, thanks to Keylabs. The platform includes every style and method of image annotation, giving developers much flexibility.
Program users may rapidly and properly categorize photographs because of its user-friendly UI. It offers a variety of capabilities to manage workflows and measure progress and enables users to work together with team members.
The software also offers viable customization options, including creating bespoke annotation templates and custom workflows. Keylabs offers built-in quality control elements in conjunction with its annotation capabilities to guarantee the correctness and consistency of annotations.
It also facilitates data augmentation approaches to improve the quantity and variety of annotated datasets. Its semantic segmentation helps solve complexities with complete ease.
Keylabs is a flexible picture annotation tool that can assist in streamlining the data annotation procedure and enhancing the precision of machine learning algorithms.
V7 Labs
The goal of V7 Labs, an image annotation tool, is to assist organizations and individuals in producing precise, high-quality datasets for machine learning and computer recognition projects. The tool’s extensive annotation feature set makes it appropriate for several situations.
The capacity of V7 Labs to provide high-quality training data rapidly and effectively is one of its primary characteristics. By accelerating the annotation process with AI-assisted annotation, the tool decreases the time and effort needed to produce accurate datasets.
TensorFlow and PyTorch are just a few of the well-liked machine learning frameworks with which V7 Labs offers seamless integration. This makes it simple for users to export annotated datasets in the style their preferred machine learning framework needs.
The tool may substantially speed up the annotation process and enhance the caliber of training data due to its collaborative workflow and AI-assisted annotation capabilities.
Labelbox
Labelbox is the world’s most potent vector labeling tool, focusing on simplicity, speed, and various use cases. It also makes the most incredible sense. Setup in minutes, scale to any team size, and quickly iterate to produce accurate training data.
It is possible to tailor the annotations to the project’s requirements, including labeling for object recognition, semantic segmentation, and image classification.

By employing dynamic filters that operate on the content, data, or text embeddings, you may quickly and efficiently add labels to appropriate results at scale and put them in a queue for human review.
With the ability to assign tasks, review notes, and keep track of progress, Labelbox facilitates cooperation between numerous users. Additionally, it offers quality assurance tools to guarantee correct and reliable labeling.
This is your go-to tool set to find and sort out the errors. You may quickly find picture data using filters like annotation to prioritize which images to label or build review assignments to address the most pressing problems.
SuperAnnotate
SuperAnnotate is a potent annotation application that enables users to quickly and accurately annotate photos and videos. To annotate datasets for computer vision models, it is made for computer vision teams, AI researchers, and data scientists.
Bounding boxes, pointers, polygons, lines, and segmentation are just a few of the many annotation types that SuperAnnotate supports.
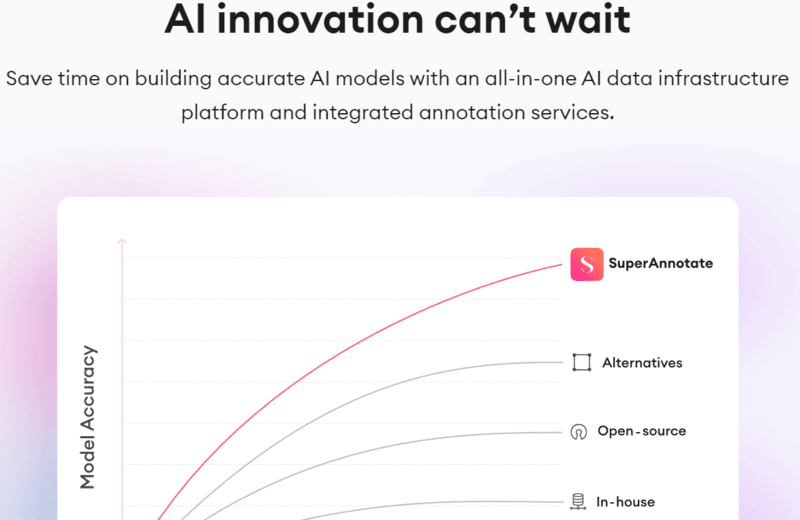
SuperAnnotate additionally offers quality control tools like auto-review and consensus review to ensure high-quality annotations. Because of the tool’s user-friendly interface, teams may work together without any problems and speed up the annotation process. It also provides an excellent query and data management feature that helps track relevant data and improves the dataset quality.
All in all, SuperAnnotate is an excellent annotation tool for data scientists and machine learning teams aiming to speed up the creation of their computer vision models, increase the quality of their annotations, and streamline their annotation process.
Supervisely
With the help of the annotation tool Supervisely, users can annotate and label pictures and videos for use in computer vision tasks. The platform’s user-friendly interface supports various annotation kinds, including object recognition, segmentation, categorization, and tracking.
With options like automatic polygonal segmentation, shape, text manipulation, and straightforward labeling, Supervisely’s robust annotating engine makes annotating easier.
Users of Supervisely can work together with other members of their team by sharing their work, making comments, and going over annotations. As a result of the platform’s integration with well-known deep learning frameworks like TensorFlow, PyTorch, and Caffe, users can also export their annotations in various formats.
Supervisely is a well-liked annotation tool among computer vision engineers, data scientists, and academics due to its powerful annotation features and effortless interaction with deep learning frameworks. In-depth lessons and documentation are also available on the site to assist customers in starting their annotation projects fast and effectively.
Scalabel
Scalabel helps people improve accuracy by using automated annotations. It is made with scalability, adaptability, and user-friendliness in mind. Several people can work on the same project simultaneously with Scalabel’s collaboration and version control support.
Additionally, it provides a range of features for quality control, including tools for review, validation, and correction.
One of the distinguishing characteristics of Scalabel is its capacity to interact with other machine learning frameworks, such as TensorFlow, PyTorch, and Caffe, enabling users to train models right inside the application. With its 3D Cloud and 2D Video Tracking, predicting annotations between frames is now seamless.
Since it provides a variety of annotation types, interaction, version control, quality control, and interaction with machine learning frameworks, Scalabel is a robust and adaptable annotation tool that is well-liked by researchers and developers in the machine learning field.
Eagle
The ideal tool for organizing images and ideas is Eagle. The tool aims to simplify the annotation of massive datasets so that computer vision models may be trained more quickly. The program also enables users to track progress, check the caliber annotations, and see and change annotations.
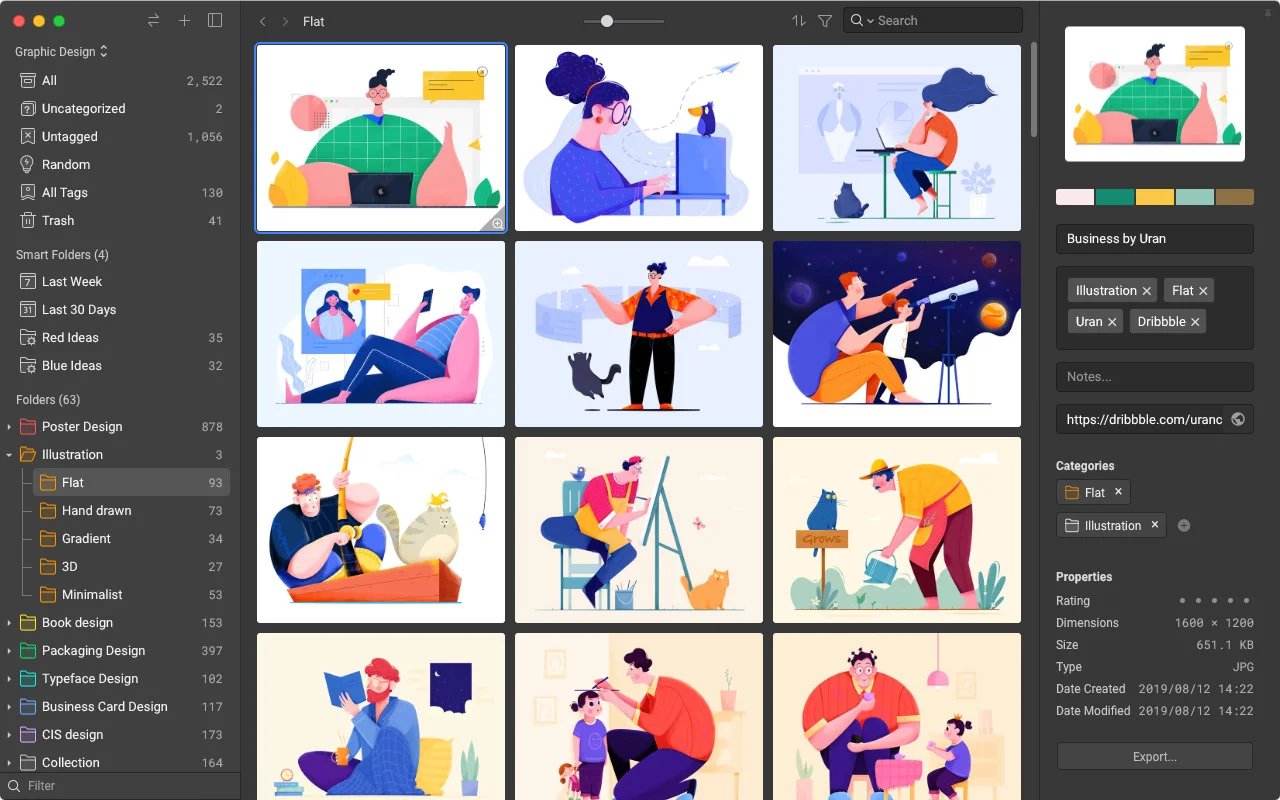
Eagle provides a user-friendly user interface and encourages teamwork. It offers several helpful features, such as organizing labeling tasks, defining annotation types, and importing/exporting datasets. Eagle also helps you to manage many images properly, enabling you to find a particular image set more quickly using the smart folder feature.
Furthermore, with its audio and video managing feature, one can preview the videos without opening them individually.
Moreover, it provides cloud service integration, making it simple to scale and handle massive datasets using Google Cloud. Ultimately, Eagle is a robust and adaptable image processing and analysis tool that works particularly well for object recognition and segmentation applications in machine learning.
RectLabel
An image labeling tool called RectLabel annotates photos for machine learning applications. Users can annotate images using this user-friendly software by drawing bounding box coordinates around exciting elements in the image. Bounding boxes, polygons, and lines are just a few of the different annotation types that the tool can handle.

Several capabilities offered by RectLabel help ensure the annotation process’s accuracy and efficiency. It also has an intelligent tagging system that expedites labeling by automatically suggesting labels based on the user’s prior annotations.
Additionally, the tool gives users precise control over the bounding box size and placement, resulting in high-quality annotations.
RectLabel enables users to extract the annotations in several different file formats, including JPG, PNG, and BMP. RectLabel is a powerful and adaptable tool for picture annotation that streamlines the procedure for machine learning jobs.
Conclusion
Global digitalization is intensifying the demand for models that use deep learning and the capability of machines to identify and categorize items. Regardless of the market, image annotation is the hub of your most excellent computer vision outcomes.
Finally, adopting image annotation techniques can benefit many businesses, such as machine learning, healthcare, and e-commerce. While doing tasks like object recognition, segmentation, and classification, these techniques can increase efficiency and accuracy.
They can also lower the cost of human labor and assist businesses in making data-driven decisions. Choosing the right tool and ensuring the annotations are high quality is essential to get the best results.
you may also explore some best AI image generators for amazing images in seconds.