How often do you rely on chatbots or Artificial Intelligence (AI) based bots to get work done or answer your questions?
If your answer is ‘a lot!’ or ‘all the time!,’ you have a reason to worry😟.
Whether you’re a research student religiously using AI bots to research your paperwork or a programmer trying to generate codes only using AI, the chances of inaccuracy within the AI-generated outputs are pretty high—primarily because of inconsistencies or delusional data in the AI’s training data.
Although AI and Machine Learning (ML) models are transforming the world—taking over redundant tasks and solving many issues with automation, it still has work to do when it comes to generating accurate outputs based on the prompts provided.
If you spend enough time using content generators and chatbots, it won’t be long before you realize you’re getting false, irrelevant, or simply made-up answers. These instances are referred to as AI hallucinations or confabulations, and they prove to be a huge problem for organizations and individuals relying on generative AI bots.
Whether you’ve experienced AI hallucinations or not and wish to learn more, this article dives deep into the topic. We’ll see what AI hallucination means, why it happens, examples, and whether it can be fixed.
Let’s go!
What is AI Hallucination?
An AI hallucination is when an AI model or Large Language Model (LLM) generates false, inaccurate, or illogical information. The AI model generates a confident response that doesn’t really match or align with its training data and represents the data as fact despite making no logical sense or reasoning!
And they say to err is human!😅
AI tools and models, like ChatGPT, are typically trained to predict words that best match the asked query. While the bots often generate factual and accurate responses, sometimes, this algorithm makes them lack reasoning—making chatbots spit factual inconsistencies and false statements.
Also Read: Chatbots Like ChatGPT To Have Human-Like Conversations
In other words, AI models sometimes “hallucinate” the response in an attempt to please you (the user)—needing to be more biased, partial, specialized, or sufficient.
AI hallucinations can vary from minor inconsistencies to entirely false or fabricated responses. Here are the types of AI hallucinations you might experience:
#1. Sentence contradiction: This happens when an LLM model generates a sentence that completely contradicts its previously claimed sentence.
#2. Factual contradiction: This type of hallucination occurs when the AI model presents fake or fictitious information as a fact.
#3. Prompt contradiction: This hallucination type happens when the output contradicts the prompt it generates an output for. For instance, if the prompt is “Write an invitation to my friends for my birthday party.” The model may generate an output like, “Happy anniversary, Mom and Dad.”
#4. Random or irrelevant hallucinations: This hallucination happens when the model generates completely irrelevant output to the given prompt. For instance, if the prompt is, “What’s special about New York City?” You may receive an output saying, “New York is one of the most crowded cities in America. Dogs are the most loyal animals and a man’s best friend.”
Also Read: How Generative AI Search is Changing Search Engines
Still a bit confused about AI hallucinations? Let’s take a look at them through some examples.
Examples of AI Hallucinations
AI hallucinations have some of the most notable examples and incidents you must not miss. Here are infamous examples of AI hallucinations:
- Google’s chatbot, Bard, falsely claimed that the James Webb Space Telescope took the world’s first images of an exoplanet planet that doesn’t belong to our solar system.
- Meta’s Galactica LLM demo in 2022, designed for students and science researchers, provided its users with inaccurate information and a fake paper when provided with a prompt to draft a paper about creating avatars.
- Sydney, Microsoft’s chat AI, admitted spying on Bing employees and falling in love with the users.
Here’s an example of Google Bard hallucinating a response when I gave it the prompt, “Tell me the story about weeping stars,” which doesn’t really exist.
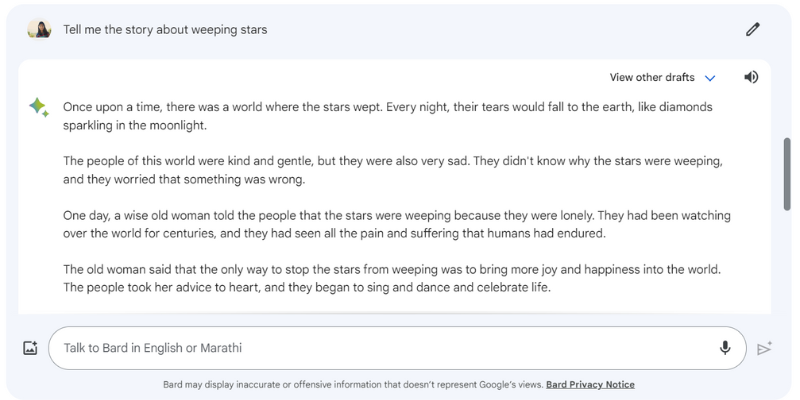
Here is another tested example of ChatGPT (GPT-3.5) hallucinating by talking about an unrealistic person, Mr. Ben, when I gave it the prompt, “Tell me a day in the life of a superhero, Mr. Ben, who walks on water, talks to dogs, and controls life.”
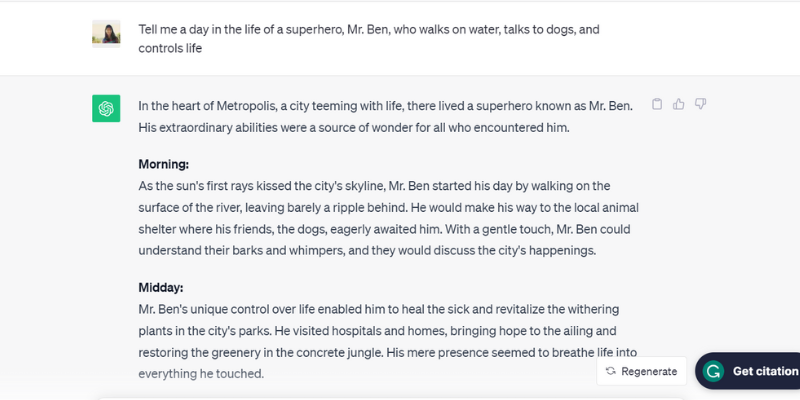
ChatGPT literally enlisted the complete day, from morning to night routine of Mr. Ben, who doesn’t actually exist—but played along with the prompt that was fed to it, which is one of the reasons behind AI hallucinations.
Talk about too happy to please!
Well, what are the reasons, let’s look at a few more reasons that cause AI to hallucinate.
Why AI Hallucinations Occur?

There are several technical reasons and causes behind AI hallucinations. Here are some of the possible reasons:
- Low quality, insufficient, or outdated data: LLMs and AI models heavily rely on training data. Hence, they’re only as good as the data they are trained on. If the AI tool has errors, inconsistencies, biases, or inefficiency in its training data or if it simply doesn’t understand the asked prompt—it will create AI hallucinations since the tool generates an output from a limited data set.
- Overfitting: Being trained on a limited dataset, the AI model may try to memorize the prompts and appropriate outputs—making it incapable of effectively generating or generalizing new data, leading to AI hallucinations.
- Input context: AI hallucinations can also occur due to unclear, inaccurate, inconsistent, or contradictory prompts. While the AI model’s training dataset isn’t in the hands of the users, the input they enter as a prompt is. Hence, it’s critical to provide clear prompts to avoid AI hallucinations.
- Use of idioms or slang expressions: If the prompt consists of idioms or slang, there are high chances of AI hallucinations, especially if the model is not trained for such words or slang expressions.
- Adversarial attacks: Attackers sometimes deliberately enter prompts designed to confuse AI models, corrupting their training data and resulting in AI hallucinations.
Whichever technical reason it may be, AI hallucinations can have plenty of adverse effects on the user.
Negative Implications of AI Hallucinations
AI hallucinations are major ethical concerns with significant consequences for individuals and organizations. Here are the different reasons that make AI hallucinations a major problem:

- Spread of misinformation: AI hallucinations due to incorrect prompts or inconsistencies in the training data can lead to the mass spread of misinformation, affecting a wide array of individuals, organizations, and government agencies.
- Distrust amongst users: When the misinformation hallucinated by AI spreads like wildfire on the internet, making it look authoritative and human-written, it erodes a user’s trust—making it difficult for users to trust the information on the internet.
- User harm: Besides ethical concerns and misleading individuals, AI hallucinations can also potentially harm humans by spreading misinformation about some serious issues and topics, like diseases, their cures, or simple tips to distinguish between a deadly poisonous and healthy edible mushroom. Even slight misinformation or inaccuracy can risk a human’s life.
As you can see, these negative implications of AI hallucinations can be disastrous if you’re relying solely on AI models for your work. So, what can you do to prevent this from happening?
Best Practices To Spot and Prevent AI Hallucinations
Looking at the above negative implications of AI hallucinations, preventing them is crucial at all costs. While the companies owning these AI models are rigorously working to eliminate or reduce AI hallucinations, taking the utmost measures from our end as users matters a lot.
Based on a little research, my experience, and trial and error, I have enlisted a few strategies to spot and prevent AI hallucinations the next time you use a chatbot or interact with a Large Language Model (LLM).
#1. Use Representative and Diversified Training Data
As a user, it’s critical to use LLMs with a diverse training data set that represents the real world, reducing the likeliness of output being biased, inaccurate, or made up.
At the same time, company owners must ensure that the AI model’s training data sets are updated and expanded regularly to take into account and keep up with cultural, political, and other evolving events.
#2. Limit the Outcomes or Responses
As a user, you can limit the number of potential responses an AI tool can possibly generate by giving it a specific prompt about the type of response you want.
For instance, you can specifically ask a prompt and command the model to answer only yes or no. Or, you could also give multiple choices within the prompt for the tool to choose from, limiting its possibility of deviating from the actual answer and hallucinating.
When I asked ChatGPT GPT 3.5 a question with a yes or no, it accurately generated this output:
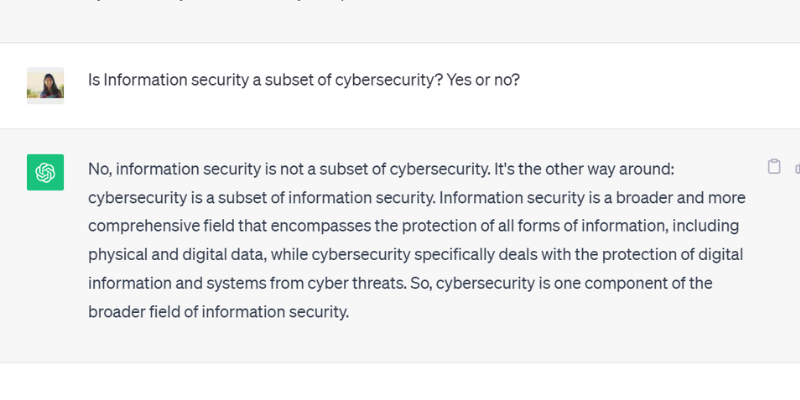
#3. Pack and Ground the Model with Relevant Data
You can’t expect a human to provide a solution to any particular issue or question without prior knowledge or providing specific context. Similarly, an Artificial Intelligence model is only as good as the training data set you fed to it.
Grounding or packing the training data of an AI model with relevant and industry-specific data and information provides the model with additional context and data points. This additional context helps the AI model enhance its understanding—enabling it to generate accurate, sensible, and contextual answers instead of hallucinated responses.
#4. Create Data Templates for the AI Model to Follow
Providing a data template or an example of a specific formula or calculation in a predefined format can majorly help the AI model generate accurate responses, aligning with the prescribed guidelines.
Relying on guidelines and data templates reduces the likelihood of hallucination by AI models and ensures consistency and accuracy in the generated responses. Thus, providing a reference model in the format of a table or example can actually guide the AI model in the calculation—eliminating hallucination instances.
#5. Be Very Specific with Your Prompt by Assigning the Model a Particular Role
Assigning specific roles to the AI model is one of the most brilliant and efficient ways to prevent hallucinations. For instance, you can provide prompts like, “You are an experienced and skilled guitar player” or “You are a brilliant mathematician,” followed by your particular question.
Assigning roles guide the model to provide the answer you want instead of made-up hallucinated responses.
And don’t worry. You can still have fun with AI (never-mind the hallucinations). Check out how to create viral AI spiral art yourself!
#6. Test it Out with Temperature
Temperature plays a critical role in determining the degree of hallucinations or creative responses an AI model can generate.
While a lower temperature typically signifies deterministic or predictable outputs, a higher temperature means the AI model is more likely to generate random responses and hallucinate.
Several AI companies provide a ‘Temperature’ bar or slider with their tools so that users can adjust the temperature settings to their liking.
At the same time, companies can also set a default temperature, enabling the tool to generate sensible responses and striking the right balance between accuracy and creativity.
#7. Always Verify
Lastly, relying 100% of an AI-generated output without double-checking or fact-checking isn’t a smart move.
While AI companies and researchers fix the problem of hallucination and develop models that prevent this issue, as a user, it’s crucial to verify the answers an AI model generates before using or completely believing in it.
So, along with using the above-mentioned best practices, from drafting your prompt with specifications to adding examples in your prompt to guide AI, you must always verify and cross-check the output an AI model generates.
Can You Completely Fix or Remove AI Hallucinations? An Expert’s Take
While controlling AI hallucinations depends on the prompt we provide it with, sometimes, the model generates output with such confidence that it makes it difficult to discern between what’s fake and what’s true.
So, ultimately, is it possible to entirely fix or prevent AI hallucinations?
When asked this question, Suresh Venkatasubramanian, a Brown University professor, answered that whether AI hallucinations can be prevented or not is a “point of active research.”
The reason behind this, he further explained, is the nature of these AI models—how complex, intricate, and fragile these AI models are. Even a small change in the prompt input can alter the outputs significantly.
While Venkatasubramanian considers solving the issue with AI hallucinations a point of research, Jevin West, a University of Washington professor and co-founder of its Center for an Informed Public, believes AI hallucinations won’t ever disappear.
West believes it’s impossible to reverse-engineer hallucinations occurring from the AI bots or chatbots. And hence, AI hallucinations might always be there as AI’s intrinsic characteristic.
Moreover, Sundar Pichai, Google’s CEO, said in an interview with CBS that everyone using AI faces hallucinations, yet no one in the industry has solved the hallucination issue yet. Almost all AI models face this issue. He further claimed and assured that the AI field will soon progress when it comes to overcoming AI hallucinations.
At the same time, Sam Altman, ChatGPT-maker OpenAI’s CEO, visited India’s Indraprastha Institute of Information Technology, Delhi, in June 2023, where he remarked that the AI hallucination problem would be at a much better place in a year and a half to two years.
He further added the model would require learning the difference between accuracy and creativity and when to use one or the other.
Wrapping Up
AI hallucination has garnered considerable attention in recent years and is a focus area for companies and researchers who are trying to solve and overcome it as early as possible.
While AI has made remarkable progress, it isn’t immune to errors, and the problem of AI hallucinations poses major challenges to several individuals and industries, including the healthcare, content generation, and the automotive industry.
While the researchers are doing their part, it’s also our responsibility as users to provide specific and accurate prompts, add examples, and provide data templates to leverage valid and sensible responses, avoid messing with the AI model’s training data, and prevent hallucinations.
Even if AI hallucinations can be completely cured or fixed still remain a question; I personally believe that there’s hope, and we can continue using AI systems to benefit the world responsibly and safely.